Interview conducted by夏娃·温赖特Feb 8 2022
In this interview, Dr. David Honigs, Ph.D, the Field Applications Scientist at PerkinElmer, Inc., talks to AZoM about the development of Honigs Regression.
Could you introduce yourself and the work that you do?
我是大卫·霍尼格斯(David Honigs),我为珀金内尔仪器(Perkinelmer Instruments)工作。当我为一家名为Perten的公司工作时,我开始开发Honigs回归,此后Perkinelmer购买了该公司。我想谈谈荣誉回归的基本思想和这种类型的分析。
I will also give some insight into how Honigs Regression works in contrast to Partial Least Squares, which is a common regression technique frequently used in many fields, including near-infrared spectroscopy. One of the things that makes Honigs Regression so attractive is how quickly it can update with new data.
Why do we make regressions in the first place?
We make regressions because near-infrared spectra are easy to measure and it is the chemistry that is the hard part. So whenever we can measure a proximate chemical constituent by near-infrared, we would like to use that near-infrared spectrum instead of the chemical measurements.
创建校准的校准或回归将NIR光谱与化学成分相关联。回归过程进行的校准既简单又便宜。我喜欢的一件事是,他们从来没有真正危害的东西。您可以在喝一杯咖啡上饮咖啡,并且不会造成任何实验室危险。
Could you talk about some of the ideas behind regression?
A regression line is sometimes called a best fit or sometimes the least-squares fit. If we plot data points and look at the absorbance at one wavelength versus absorbance at another, you can see the best line that goes through them. That line is often called the regression line.
如果我们有一个以上的维度,那么我们以多个维度的回归结束。因此,我们没有找到最佳的线,而是找到适合两个变量之间的最佳平面。因此,对于那些不同的变量,基本上预计样品浓度是在该平面上。
As we add more dimensions, we would go from that line with one dimension, comparing two things to a plane, comparing three things to a cubic space, comparing more and more, and higher and higher geometries.
这在回归中的变量方面意味着什么?
因此,多个线性回归使用了多个变量。多线性回归通常使用所谓的线性模型。我们期望随着一切的增加,随着线路的加倍,浓度加倍,我们的信号与这种变化成正比。非线性增加将是其他一些模式,例如指数。数据拟合已知模式,但其增长率与直线不同。
The fact is that when we deal with regression and near-infrared spectra, we use this linear assumption. It is embedded in Partial Least Squares, for example, and there is linear technique underneath everything. But the world tends to be non-linear and so we are then left trying to take our linear model, our PLS and try to fit it into a non-linear situation. When that happens, the results of the regression and the calibration line are not going to fit the data as well as we might like.
那么如何在校准线和数据之间解决此问题?
我想介绍的另一个概念是哑铃分布的想法。我们称之为分布,因为它们倾向于在几个斑点上有很多样本,而间隔不多。
These have what you call a within-group relationship. It turns out that a dumbbell distribution is exceptionally hard for PLS to handle well, and it comes up all the time. It especially comes up with factories that are making defined products that are related to each other.
这些是我们校准面临的基本问题。我们在PL中使用的多个线性回归中使用的大多数工具都是线性的,并且自然不是线性的。很多时候,我们都有一组没有样本的产品组,而回归很难决定是否要解释组间距离或是否要解释组内差异。
How do you resolve the issue of dumbbell distribution?
如果您想拥有一个非常好的相关系数,那么当您获得一些非常低的样本,而有些样品很高,则最终的回归线将很长。但是,r平方或r是您正在看的,这将是重要的数字,但这并不意味着它实际上是在解释任何内容,因为所解释的大部分只是两组之间的区别,而不是什么在这两个群体的内部进行。
Once the calibration has been built and the regression work carried out, it is worth noting that the regressions need to be updated year after year when the process changes, when supplies change and when crop years change.
正是因为事情不断变化,校准不是通用的。校准是用一定的样本进行的,一旦我们走出那组样本及其代表的内容,事情就会改变。这些是我年复一年地面对的问题。
How do you get out of the trap of having to do these models over and over and over?
If you hold yourself to a mathematician's level of truth, you can build some amazing things that are based on the truth that will always help you. They may not solve your exact problem, but at least you can be confident in the tools that you build with this type of approach.
One thing I can say is that if the spectrum is the same, the concentration must be the same. Every single equation out there, whether it is PLS or ANN or anything else, has this property. We would say that this problem is one to one. This spectrum has this answer, and a different spectrum may have a different answer. The problem is ideally one-to-one.
The real question is, are the spectra actually the same? And the answer is that they are rarely even close.
We have to figure out how to handle the spectra to get rid of any differences. It is fundamental to Honigs Regression that we deal with spectra where this is true. If the spectrum is the same, the answer is the same. It is called a previously solved problem. If we have that previously solved problem, how do we make that more true?
That is the first job and the way we do that is with pre-treatments. So if instead of the spectrum being the same, we now change this to say, if the pretreated spectrum is the same, then the concentrations must be the same. We are saying that it is okay to filter out things like scatter and particle size that are not important to the problem and try to work on this reduced problem.
How do pretreatments help in relation to the spectrum?
Some pre-treatments do not remove all of the undesirable things and so we are still left with many variations that give the same answer, and some actually change the information that is the real spectrum.
When they do this, they are also going to potentially change our answer. So the pre-treatments that do this, that will take and remove the scatter, will take and remove the baseline shift. Those types of things must be more useful as data treatments than the others. Data treatments that demonstrate this behavior are standard normal variant, de-trending, mean centering, orthogonalization, similar sorts of idempotent functions
By returning to the first principle, there is this idea of a pretreatment that we are going to use that will change the spectrum to the point where it is identical to other spectra of this same material. That has to do with instrument matching, particle size, scattering effects, and optical geometries. That is a really important step to understanding this.
回归中发生的另一件事是,平均值成为最确定的位置。当考虑最合适的线时,我们的错误是在回归线中。您看到的我们的不确定性有腰,腰部正当。因此,这是最确定的位置,当那里有样本时,我们知道它的浓度必须在该线上,再加上最少的数量。
Instead of thinking of regressions as just a bunch of data points with the line through them, we think about regressions as having uncertainty to them and that the most certain spot is the mean. As a matter of fact, it is said that if you do not know anything other than the mean if you guess the mean you are going to be as close as possible in that circumstance.
How can we make use of this?
One thing that has been tried previously is cascading calibrations. We have one calibration that possesses a certain accuracy over a large range. And then we break that into two calibrations, which will handle the separate ranges. And then we could even break those two into two more.
We wind up with a total of four separate calibrations. Our answer essentially pings off of the first one, into the second one, which picks which of the four we should use, which gives us the result. People have been doing this for at least 30 years in the field of near-infrared, and probably much longer than that in other areas.
This process handles those dumbbell relationships, and the first calibration is going to separate it into which group it belongs to in the dumbbell. The next one is going to be focused on one end or the other, and that will help it better fit the between-sample variability instead of the between-group variability. So this is a useful technique.
为什么人们不再使用此技术?
People tend not to use this technique as much as it gets harder and harder to make and maintain so many calibrations. If you have a lab error when you are trying to decide which sample you should use to make the calibration, there is a limit as to how many of these divisions you can make. You can definitely divide things into two. Sometimes you can divide them into four. After that, it really depends on the accuracy of your lab technique and the variability of your data.
Instead of using separate calibrations, each depicting the next calibration and so on, I could group the data by the spectrum.
我知道,如果它具有相同的光谱,则必须给出相同的答案。因此,我在这里采取的飞跃是,如果它具有几乎相同的光谱,则应该有类似的答案。他们至少应该关联。
If we just keep cascading spectra, these answers get better, but there are more calibrations to make. I have seen people do this when accuracy is critical, but I have not seen very many people maintain that over time. It just becomes too difficult.
Is there a way to maintain calibrations that do not cause too many problems in the long term?
There are ways that we could break up the different regression lines, we could ask the operator which value it is.
We are asking the operator what the answers should be beforehand and then the remaining calibration just touches it up a little bit. This is how it is actually done, and I have seen this done in many different places. Operators tend to call these channels.
When you see what is called channel creep, you start to see a whole bunch of calibrations measure the same things, varying slightly only on what slight product differences are. They are essentially asking the operator what the answer should be and then giving it back to them. You wind up with many separate products, many separate biases and chasing these biases becomes a full-time job.
然后,您得到错误的答案,没有警告说这是错误的答案。该仪器没有任何方法可以知道该其他信息是否准确。正如我之前说的,您可以级联校准,您需要进行一个校准来统治它们,然后选择高低的低和低和分开它们,然后再分开它们。您必须维护所有这些。
If your lab has a significant amount of error to it, it gets harder and harder to decide which calibration the sample should be used to make. That is the limit on this approach. If the spectra look the same, maybe they are similar. So we are taking our rule of ‘same spectrum same answer’ to include similar spectra similar answers.
Is Honigs Regression better than the other calibration techniques?
We have done enough work to say that the Honigs Regression can compare reasonably well to ANN calibrations in quite a few different situations. It is not always better, but it is not necessarily worse either. The advantage that the Honigs Regression has compared to ANN or even PLS is that it is really quite easy to create, and is especially easier to update. That ease of update happens when you take essentially a sample and add its spectrum to the library.
For every spectrum that you add, you need to add its lab value for the concentrations you are interested in. So as we add new labs and new concentrations to the library, that automatically updates the Honigs Regression. It does not recompute the calibration, it is using the same calibration and adjusts how it calculates the sample means based on the new examples that it has. This makes it very easy to adapt to new situations without throwing out everything that you have done before.
As it can be updated, Honigs Regression is what is called a learning regression, which means we have a library of spectra and labs from previous examples. If we ever see that same exact spectrum again, we know that it is that same laboratory. It is kind of like a ‘reference table’ in a way.
当我们收集新的光谱和新实验室并不断扩展该库时,我们将有更多示例可以比较它。随着我们继续扩展图书馆,我们有更多的细节。扩展图书馆的是进化学习,称为缓慢的学习过程。我们不会带来革命并推翻以前所做的一切。我们只是不断使我们的东西变得更好,更好。
I like the improvement in accuracy that Honigs Regression offers. I like that it is pretty simple to do, but in my opinion, learning is the key. That is because keeping calibrations up to date and changing biases and adding a few more calibrations from a new season, is a lot of the workload that an application specialist has to do.
Some examples of learning that I have seen include a single calibration made for use on whole wheat that can also work for ground wheat, barley, malted barley, flour, and other bakery mixes. So this learning does exactly the opposite of channel creep, instead of breaking out the data into more and more calibrations that we have to maintain separate biases on, if not separate calibrations on, we wind up being able to put more and more diverse things together into one calibration.
仅进行一次校准会改善近红外测量技术吗?
The closer you come to one master calibration, the closer you come to making near-infrared a primary measurement technique instead of a secondary technique. You are getting to the fundamental cause when you relate the spectrum to the laboratory measurements.
Honigs回归从高距离的样品中学习。也就是说,那些不太适合模型的样品或具有较高全局H的样品。这些样品不是其他许多样本。一旦添加到图书馆,它就会向他们学习。
Now, when most people are making regression, the temptation is to throw those high M distance or high global H samples out and make the regression without them because they tend to cause PLS to misbehave and pay more attention to the between-group distance than it does to the within-group variability.
The Honigs Regression does not do that. It can have those samples in the library without having them make the calibration change. You do not have to update the calibration. When you get these samples, you just add them to the library. That means that you can have a tested and trusted calibration that you are very confident in and that it will adapt to local conditions and unusual samples just by adding a few more examples of them.
Basically, the Honigs Regression never stops learning. You can keep updating it and redoing the calibration for it.
How do you maintain a fixed calibration when the Honigs Regression is being persistently updated?
When talking about updating, the question that comes up in regard to maintaining calibration is biases. When noticing a change in calibration bias, it is necessary to update the bias and ask what is that bias fixing? It is likely fixing differences between the labs that the dataset was calibrated on and that you are comparing it to now. That bias is fixing differences between instruments or it is fixing differences in the same instrument over time.
在频谱中您有一个新变量的情况很大,有些事情已经变成了配方,平均而言,您可以说它会导致偏见。您将这种偏见放在其中,校准将继续运行。客户会很高兴,但您确实没有解释正在发生的事情。
That is because when you treat the spectra, you treat them all as one group. You do not look at the separate different types of groups that are going on that cause a sample to be biased. The key is using data to adjust the bias without messing with the data that you use to adjust these samples.
To me, the idea of fixing a bias means that something is wrong with your calibration or your instrument or your laboratory. It does not mean that you fixed anything. It means that you have covered something up. If you were adding samples to the library and the library adapts, that is actually fixing something, that is getting the cause in the data set.
Could you perhaps give us an example of how this could work in an application setting?
当对一种类型的材料进行校准来预测另一种材料时,我们不会期望它可以很好地运行。因此,当我们在反刍动物测试集中使用反刍动物校准时,我们可以看到支持矢量机和荣誉回归几乎具有相同的精度。
In this case, the support-vector machine is just a little bit lower than the Honigs Regression.
The PLS is a good technique. It is giving a reasonable answer, but PLS is just not as accurate as HR or support-vector machines on complicated problems or complicated distributions.
When we use these calibrations to predict our monogastric feeds, the support-vector machine is not a good choice, but it does significantly better than either the PLS or the Honigs Regression. As we would expect, if we take a calibration and we use it on something that is not at all like what it was calibrated for, and there is nothing like that in the library for Honigs Regression, it does not do very well.
When adding monogastric samples to our ruminant dataset, we recompute the support-vector machine calibration. We recompute the PLS calibration.
对于Honigs的回归,我们没有重新计算基本的反刍校准。我们只是将样本添加到库中。有了10个添加的样本,我们在单胃istric中的性能没有改变,除了Honigs回归以外,我们没有任何改进。荣誉回归已经开始缓慢的适应过程。
It is important to note that as you add more things to PLS, the calibrations can get worse. Because you are adding that non-linearity, you are adding that dumbbell distribution, PLS does not handle that well. As we added more samples, PLS is getting better at the monogastric because those are in there, the calibration is recomputed. They start to have some impact, but it that improvement comes with the price of degrading the accuracy with the ruminant. To make the one better, the other one has to get worse. Those two things are linked because it is a linear system.
By this time, with 100 added samples, you can see the Honigs Regression has adapted really well.
What about lab errors, how do these impact the process?
Lab errors come quite rapidly. When adding more and more samples, you would both the ruminant and the monogastric calibration data in ANN, and we make one calibration. The ANN can do pretty well on either type of material. The errors are not identical because the lab errors are different on those types of materials as well.
但是,随着Honigs的回归,它很久以前就对反刍动物数据进行了一个校准,并且只是使用这些添加的实验室样本来变得越来越好。即使我们试图预测的材料与我们的初始校准并不完全相同,学习也会不断推动我们的错误。
With PLS, there is a maximum number of samples, and once you have hit that number, things start to get worse. It is not that there is some magic number that PLS cannot handle. The math just does not behave like that.
发生的事情是,如果您添加越来越多的不同材料样本,请继续妥协。欧洲杯足球竞彩如果我们将反刍动物和单次胃汇合在一起,那么PL会比我们分开的情况更糟。
Why is Honigs Regression useful for near-infrared spectroscopy.
世界绝对不是线性的。话虽如此,分组是好的。当我们尝试将类似的东西放在一起时,我们的学习能力将提高,这将简化问题。我想补充一点,学习总是有助于解决问题。
It is the non-linear approach that makes Honigs Regression very powerful. It is the learning that frankly saves so much time as users do not have to keep adjusting biases on calibrations or keep updating calibrations. Samples can be added to the library and they are good to go.
学习也比答案更有价值。学习是数据中许多不同事物的汇编。我们永远不会完全用一种材料来完成,这并不是事物的工作方式。我们需要有能力从新数据,新样本中学习,这是为了提供正确答案而无需抛弃一切并重新开始的智慧。
About Dr. David Honigs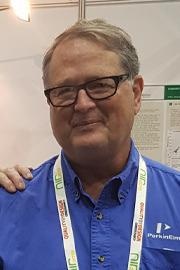
Dr. Honigs did his graduate work under joint supervision of Professor Gary Hieftje and Dr. Tomas Hirschfeld at Indiana University in Bloomington. He served as an Assistant Professor of Analytical Chemistry at the University of Washington for a few years. Following that he worked at NIRSystems (now part of FOSS) on NIR instruments. He started a company, Katrina Inc. which made process NIR instruments.
For the last almost 20 years he has worked at Perten (now Perkin Elmer) on Near Infrared Instrumentation and applications in the food industry. He has 35 research papers as listed on ResearchGate.com He has 10 issued US patents.
About PerkinElmer Food Safety and Quality
PerkinElmer Food Safety and Quality is committed to providing the innovative analytical tools needed to ensure the global supply of high-quality, safe and unadulterated foods.
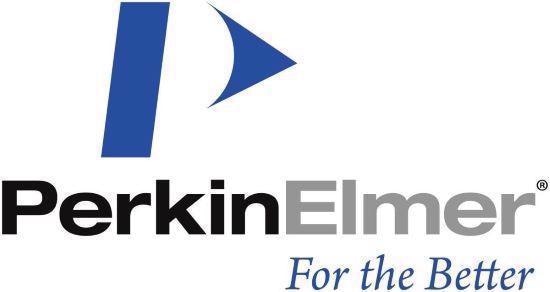
此信息已从Perkinelmer食品安全和质量提供的材料中采购,审查和改编。欧洲杯足球竞彩
For more information on this source, please visitPerkinelmer食品安全和质量。
Disclaimer: The views expressed here are those of the interviewee and do not necessarily represent the views of AZoM.com Limited (T/A) AZoNetwork, the owner and operator of this website. This disclaimer forms part of theTerms and Conditionsof use of this website.